Content Moderation in a New Era for AI and Automation
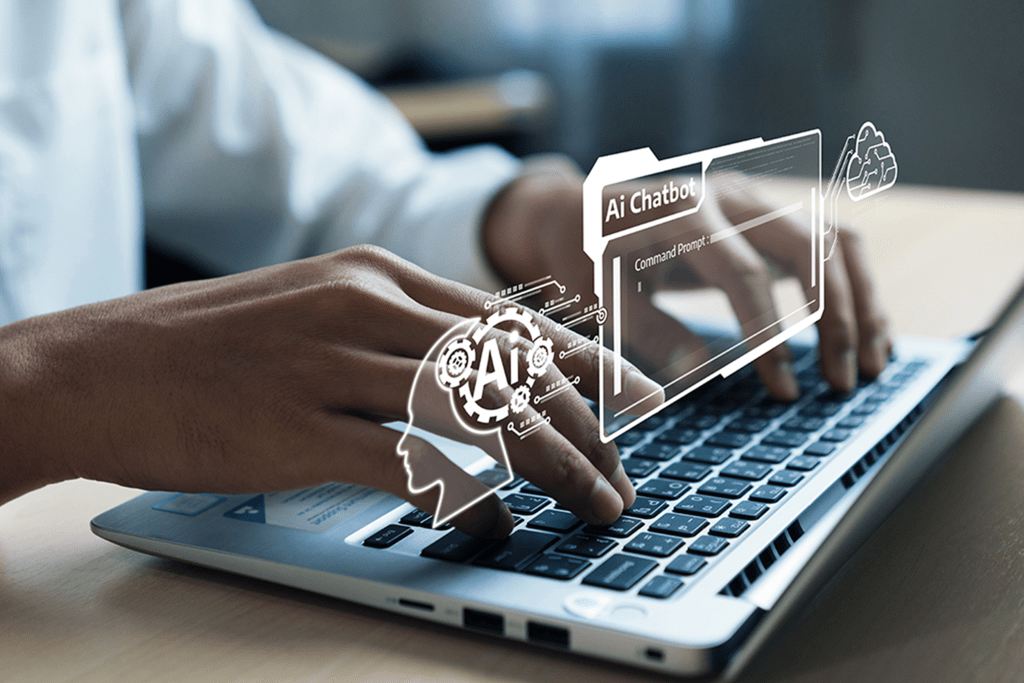
Introduction
The ways in which social media companies enforce their content rules and curate people’s feeds have dramatically evolved over the 20 years since Facebook was launched in 2004. Today, automated classifiers parse through content and decide what should be left up, taken down or sent for human review. Artificial intelligence (AI) systems analyze users’ behavior to tailor online experiences by ranking posts.
Meanwhile, the quality of tools used by people around the world to create and alter content has significantly improved. From autocorrect on a phone keypad to face filters, video editing and generative chatbots, tools for user-generated content are remarkably more sophisticated compared to when social media started.
These developments represent a major shift impacting billions of people on social media. The mass availability of powerful new tools has profound implications, both for the decisions that companies make to design, develop and incorporate these technologies into their products, as well as the content policies enforced against higher quality user-generated content.
Most content moderation decisions are now made by machines, not human beings, and this is only set to accelerate. Automation amplifies human error, with biases embedded in training data and system design, while enforcement decisions happen rapidly, leaving limited opportunities for human oversight.
AI algorithms can reinforce existing societal biases or lean to one side of ideological divides. It is imperative for platforms to ensure that freedom of expression and human rights considerations are embedded in these tools early and by design, bearing in mind the immense institutional and technological challenges of overhauling systems already operating at a massive scale.
The Oversight Board, an independent body of 21 human rights experts from around the world, has investigated emblematic cases involving how Meta’s content policies are enforced by AI algorithms and automation techniques. The Board’s human rights-based approach goes far beyond deciding what specific content should be left up or taken down. Our cases delve into the design and function of Meta’s automated systems to shine a light on what factors lead to content moderation decisions, and how those tools can be improved.
These cases explore key issues such as automated content removal systems, including what Meta calls Media Matching Service banks; policies for AI-generated explicit images and other manipulated media; and how AI and automated systems struggle to understand context, leading to incorrect applications of the rules. By leveraging our portfolio of casework, ongoing engagement with civil society and the areas in which the Board has successfully enacted change across Meta’s platforms, this paper shares our key lessons for industry, regulators, experts and users at large.
Key Lessons for Industry
- To help confront the proliferation of deepfake intimate images on social media, platforms should focus their policies on identifying lack of consent among those targeted by such content. AI generation or manipulation should be considered as a signal that such images could be non-consensual.
- Platforms should leverage automation to empower people to better understand policies and prevent erroneous removal of their own content, including through informative user notifications. People deserve an explanation as to why their content was taken down and whether this was a human or automated decision. When appealing content that was taken down, people should also be given opportunities to provide context about their post that content moderators, whether human or AI, may not have correctly interpreted, for example satire, awareness raising and condemnation. The Board has pushed Meta to launch new features to this end, which are already helping millions of users.
- The benefits of new generative AI models should be shared equitably by social media companies’ global user bases – beyond English-speaking countries or markets in the West where platforms typically concentrate the most resources. These improvements may include greater transparency, more accurate accounting for context and identifying violations on a more granular level. This is especially important as low language and context competence can lead to over- and under-enforcement.
- Automated moderation and curation systems must be rigorously and continually evaluated on their performance for users who are most vulnerable and most at risk. As new models are deployed, it is especially important to ensure they do not exacerbate existing societal biases that may adversely affect marginalized groups and others.
- Global human rights, freedom of expression and ethics experts should be consulted when designing and deploying new AI-powered content moderation tools early in the process. Risk mitigations and other product guardrails, recommended by such experts, should be incorporated into their design.
- Transparency is paramount. Third-party researchers, from around the world, should be given access to data allowing them to assess the impact of algorithmic content moderation, feed curation and AI tools for user-generated content.
- Information can help address misinformation and disinformation. Platforms should apply labels indicating to users when content is significantly altered and could mislead, while also dedicating sufficient resources to human review that supports this work.
CHALLENGES FOR MODERATING CONTENT IN THE GENERATIVE AI ERA
There are many reasons to be excited and optimistic about generative AI. It has undoubtedly delivered benefits to content creators and businesses, from better photo editing capabilities to language translation and customer service chatbots.
As the American Civil Liberties Union (ACLU) pointed out in a public comment to the Board, not all manipulated media is inherently harmful: “To the contrary, there are uses of manipulated media that add value to public discourse – including parody and satire...as well as humorless, avowedly false speech that is nevertheless illustrative or thought-provoking...” Platforms have a responsibility to protect such speech.
However, generative AI, including large-language models designed to create text, audio and images, can and does contribute to existing harms on the internet, for example image-based sexual abuse or content misleading people about how or when to vote. Perhaps the most threatening aspect of these new AI-powered tools is the ease of production, which facilitates both quality and quantity. Deceivingly realistic content can be generated within seconds and without significant expertise.
While people are using AI to create content, platforms are using it to moderate content. As this new technology is deployed, social media companies should monitor whether these tools contribute to existing imbalances that undermine civil society. Researchers have posited that content moderation could potentially be improved through the use of new generative AI tools. However, this could mean that platforms use generative AI models to solve content moderation issues that are sometimes exacerbated by generative AI.
These systems will have to prove themselves on key features where previous models have struggled, such as discerning cultural and linguistic nuances in content. Data access for third-party research is critically important for understanding how these systems are performing. Potential solutions have been proposed to allow civil society to assess the underlying biases fueling these generative AI tools, which becomes even more important as these systems are adopted for content moderation.
Image-Based Sexual Abuse
Image-based sexual abuse is not new, but the explosion of novel generative AI tools to enable it marks a new era for gender-based harassment. For little or no cost, any individual with an internet connection and a photo of someone can produce sexualized imagery of that person, which can then be spread without their consent or knowledge. Researchers of online sexual abuse suggest the harms of deepfake intimate imagery may be as severe as those associated with authentic sexual images that have been shared without consent.
The overwhelming majority of this content targets women and girls, ranging from teenagers to politicians and other public figures, including celebrities. In a public comment to the Board, the Center for Democracy and Technology noted that deepfakes targeting women in politics are “meant to challenge, control, and attack their presence in spaces of public authority.”
Meanwhile, the proliferation of deepfake intimate imagery as a form of teenage bullying raises serious mental health concerns for girls. The New York Times reported on how deepfake images have grown as a form of harassment that can lead to severe emotional harm, damage reputations and threaten physical safety. One prominent case involved a student from a high school in the United States (U.S.) being targeted by classmates.
Experts consulted by the Board have also warned that this content can be particularly damaging in socially conservative communities. For instance, an 18-year-old woman was reportedly shot dead by her father and uncle in Pakistan’s remote Kohistan region after a digitally altered photograph of her with a man went viral.
A public comment from the Indian NGO Breakthrough Trust explains that in India, “women often face secondary victimisation” when accessing police or court services by being asked why they put pictures of themselves on the internet in the first place – even when the images were non-consensual deepfakes.
In July 2024, the Board issued a decision on two cases involving AI-generated and AI-manipulated images of nude women, one resembling an Indian public figure, the other a U.S. public figure. While Meta had removed the post involving the U.S. public figure from Facebook, it did not remove the post from India until the Board selected the case. In this context, removal is warranted to protect individuals from the sharing of sexual images made without their consent. The Board noted that labeling deepfake intimate imagery is not sufficient because the harms stem from the sharing and viewing of these images, not solely from misleading people about their authenticity.
Of concern, the image resembling an Indian public figure was not added to a Media Matching Service bank (more details below) by Meta until the Board asked about it. Meta responded by saying that it had relied on media reports to add the image resembling the U.S. public figure to the bank, but there was no such media coverage in the Indian case. This is worrying because many victims of non-consensual deepfake intimate images are not in the public eye and are forced to either accept the spread of such depictions or search for and report every instance.
Although media reporting may be a useful signal that this type of content is non-consensual for public figures, that is not helpful for private individuals. Therefore, social media companies should not be over-reliant on news coverage. Platforms need to be clear in their policies about what signals of non-consent would lead to removal of this type of content and ensure there are convenient pathways for users to report it.
The Board’s cases suggest that social media companies should focus their policies on the lack of consent and harms of such content proliferating. With this focus in mind, context indicating the nude or sexualized aspects of a post are AI-generated or otherwise manipulated should be considered as a signal of non-consent. Setting a standard that AI generation or manipulation of intimate images are inherently indicators of non-consent would be major step forward given the rapid increase of deepfakes.
Ultimately, social media platforms must quickly identify and remove this type of content while also making it easy for users to report it. Both India and the U.S. have considered laws and announced further plans to regulate deepfakes. However, the Board received many public comments emphasizing how important it is that platforms be the first line of defense because legal regimes may not move quickly enough to stop this content from proliferating.
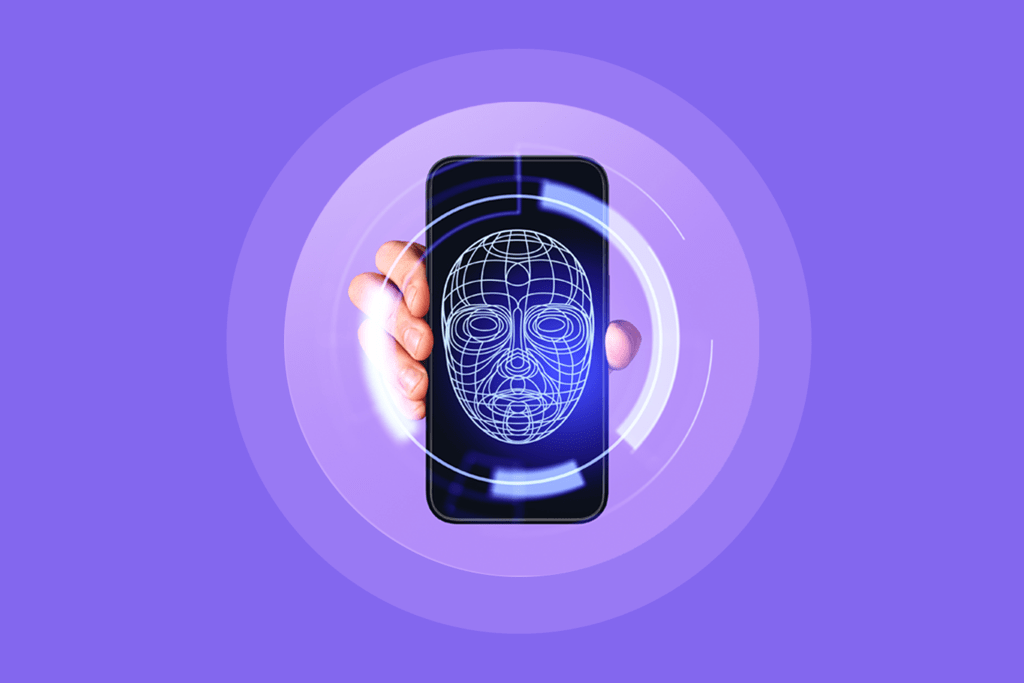
Elections
While it has been suggested that more traditional uses of AI, such as ranking algorithms, contribute to political polarization, the rise of generative AI opens new avenues for abuse during elections.
In Taiwan, deepfake audio surfaced on YouTube of a politician endorsing another candidate, which never happened. In the United Kingdom, fake audio and video clips targeted politicians from across the political spectrum. In India, where more than half a billion voters went to the polls for the 2024 elections, people were reportedly bombarded with political deepfakes, including fake endorsements from celebrities and deceased politicians.
The Board has investigated a case concerning a manipulated video of U.S. President Joe Biden, in which footage of him placing an “I voted” sticker on his granddaughter was doctored to make it appear as if he was inappropriately touching her. Of note, the video in the Biden case was not altered by AI, but rather by looping the moment the president’s hand makes contact with his granddaughter’s chest.
That the content was altered by more primitive editing tools underscores how the variety of technologies available – whether generative AI or something else – makes the precise method of manipulation less important than the risk that viewers will be misled. As such, social media companies should orient their content policies to protect against the harms they seek to prevent, rather than focusing on the technology used to produce content.
In that case, the Board also found that in some instances platforms could prevent the harm to users caused by being misled about the authenticity of content by attaching a label. Labels empower people with context, allowing them to come to their own conclusions. This is also a less intrusive approach than removals, so more content can be left up, allowing social media companies to protect users’ free expression.
Following the Board’s decision, Meta announced plans to begin labeling a wider range of images, videos and audio altered by AI. This is a clear recommendation that other platforms should consider adopting.
Language Disparity
With new generations of AI being deployed by social media platforms, it is essential companies ensure this technology can serve people fairly. Our investigations have found that content moderation resources are not always equitably distributed. For example, in the Board’s policy advisory opinion on COVID-19 Misinformation, stakeholders highlighted how most languages, besides English, have significantly less fact-checking coverage. Again, in another case concerning news reporting on the Taliban the Brennan Center for Justice expressed concern in the following public comment: “Meta’s automated tools time and again fail to account for context, particularly in languages other than English.”
Language disparity is a chief concern as platforms look to onboard large language AI models. Some tech companies are reportedly leaning into a language-agnostic approach in their large language models, owing to limited training text for certain languages. According to developers and proponents of these multilingual models, they are able to leverage their performance in some “high-resource” languages to compensate for a relative lack of “low-resource” language training data.
However, critics of these multilingual models point to potential disparities between high- and low-resource languages in terms of accuracy of detecting and enforcing violations. Despite advancements in AI-powered translation technology, it remains unclear how effectively a model trained primarily on machine-translated English can account for cultural or humorous nuances in, for example, Amharic, which is spoken by tens of millions of people in Ethiopia.
Regardless of how they are built, if new AI models are to deliver on the promise of more accurate and transparent enforcement, those benefits must be appropriately distributed across platforms’ global user bases. Companies must not evaluate model performance based solely on the results of English-language benchmarks, or of aggregated tests in which English is disproportionately represented, but rather with the breadth of their global audiences in mind.
HOW AUTOMATION GOVERNS PLATFORMS
Platforms are increasingly relying on automation for content moderation. This means automated systems, by enforcing policies, and identifying and recommending content, are deciding what humans do or do not consume as social media users.
To be clear, when discussing automation, this includes tools that are rule-based and stick to repetitive tasks like flagging posts with certain words or blocking users who repeatedly violate the rules. Comparatively, AI content moderation tools are more adaptable. They use machine learning and can attempt to make decisions based on analyzing patterns.
The upside to automation is scalability, but the concerns (at least for now) are whether these tools can balance scale with precision and prevent systemic biases. This balance is a chief concern often raised to the Board by civil society organizations and individuals.
Missing Context: How Machines Cause Over- and
Under-Enforcement
Over-Enforcement:
Without regular audits and retraining, machine classifiers can often be a blunt enforcement tool. In one of its earliest cases, the Board looked at a picture posted to Instagram to raise awareness about symptoms of breast cancer. The image was pink, in line with “Pink October,” an international campaign popular in Brazil to raise breast cancer awareness. Eight photographs within a single picture showed breast cancer symptoms with corresponding descriptions such as “ripples,” “clusters” and “wounds.” Five of the photographs included visible and uncovered female nipples. The remaining three included female breasts, with the nipples either out of shot or covered by a hand.
Despite numerous signals indicating the harmless and informative nature of the post, it was detected and removed by a machine learning classifier trained to identify nudity in photos. Meta’s Community Standards generally prohibit uncovered female nipples, but there are allowances for “educational or medical purposes,” including breast cancer awareness. Unfortunately, Meta’s automated systems failed to recognize important context, including the words “Breast Cancer” that appeared at the top of the image in Portuguese.
The Board advised Meta to improve its automated detection of images with text-overlay to ensure that posts raising awareness of breast cancer symptoms are not wrongly flagged for review. In response, Meta enhanced Instagram’s techniques for identifying contextual signals, including through text, that are relevant to breast cancer. The company deployed these changes in July 2021, with these enhancements in place since. To give a snapshot of the impact from these improvements, in the 30 days between February 26 and March 27, 2023, these enhancements contributed to an additional 2,500 pieces of content being sent for human review that would have previously been removed.
Given the volume, scale and speed at which content is spread on social media, the Board accepts that automation is essential to the detection of potentially violating content. However, enforcement that relies solely on automation, when using technologies with a limited ability to understand context, can lead to over-enforcement that disproportionately interferes with freedom of expression.
To be clear, automation works for a large portion of content moderation but often fails in niche, critically important situations like the previously explained example. Automation could be better at understanding context, but it takes oversight and resources to finetune these tools like in the breast cancer case. With new generations of AI and automation, platforms should commit to refining the quality of enforcement against important themes of content (for example, health education) and where there are high rates of enforcement errors.
- Penalties: The Board is also concerned about the penalties associated with over-enforcement by automation. Posts can be wrongly removed by automation, with the relevant accounts sanctioned or their content demoted. An account’s violation history can determine whether more severe penalties are imposed, including posting restrictions. Because automation moves so quickly, violations can pile up and disable accounts. The Board has had success in pushing Meta to reform its strikes system, including through new notifications explaining why content was removed and by providing greater transparency about the system and its penalties. However, there is more room for improvement around the most serious violations, which can severely impact journalists and activists. That is why the Board has asked for greater transparency on “severe strikes” and will continue to do so.
Under-Enforcement:
Coded language is nothing new or uncommon. On the internet, phrases like “unalive” can mean death, anti-vaccine Facebook groups are called “dinner parties,” and sex workers are referred to as “accountants.” Users often intentionally misspell words (c0vid) or use emojis, such as watermelon slices when referencing Palestine to evade algorithmic detection and enforcement.
But when hate speech is coded to evade detection from automated systems, it can contribute to an unsafe online environment.
The Wilson Center, a Washington D.C.-based think tank, refers to coded hate speech as “malign creativity,” and says it is the greatest obstacle to detecting and enforcing against gender-based attacks online. It can come in the form of satire or context-based visuals that require situational knowledge to understand, and automated tools usually aren’t calibrated to detect them.
In the Board’s Post in Polish Targeting Trans People case, the Oversight Board overturned Meta’s original decision to leave up a Facebook post in which a user targeted transgender people with violent speech advocating suicide. The post contained an image of a striped curtain in the blue, pink and white colors of the transgender flag, with text in Polish. Meta’s automated systems failed to notice key contextual clues, including a reference to suicide (“curtains that hang themselves”), support for the death of trans people (“spring cleaning”) and even a self-admission in the user’s bio that they are transphobic.
The fundamental issue in this case was not with Meta’s policies, but its enforcement. Automated systems that both enforce content policies and prioritize content for review need training to be able to recognize the kind of coded language and context-based images considered in this case. It is critically important that platforms audit the accuracy of these systems, particularly in regard to coded references.
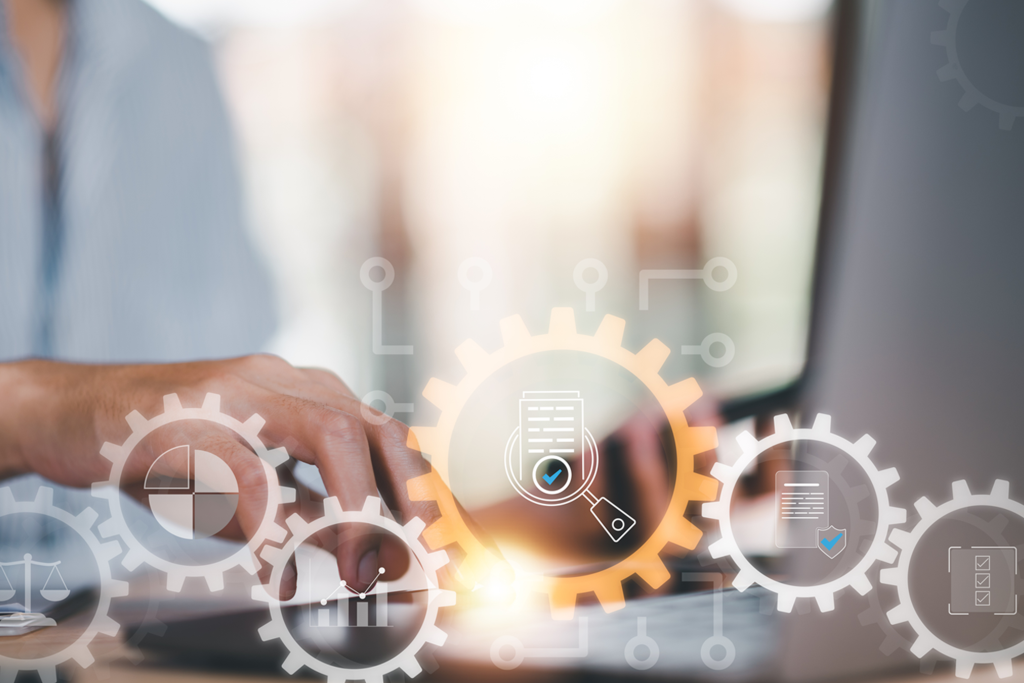
Case Studies
The Board is more than three years into issuing decisions, and has begun to better understand the impact of its recommendations on users once they are implemented. The two case studies below present data demonstrating how changes the Board pushed Meta on implementing allow users to either add context that automation may have missed or edit their post before a potential automated removal decision is made.
Allowing users to provide context
People often tell us that Meta has taken down posts calling attention to hate speech for the purposes of condemnation, mockery or awareness raising because of the inability of automated systems (and sometimes human reviewers) to distinguish between such posts and hate speech itself. To address this, the Board recommended that Meta create a convenient way for users to indicate in their appeal that their post fell into one of these categories. Meta agreed to this and the feature is already seeing strong engagement from users.
In February 2024, Meta received more than seven million appeals from people whose content had been removed under its rules on hate speech. Eight out of 10 of those appealing chose to use this new option to provide additional context. One in five of these users indicated that their content was meant “to raise awareness,” while one in three chose “it was a joke.” The Board believes that giving people a voice – and listening to them – can help Meta make better decisions.
Alerts that empower users to make their own decision
In the Pro-Navalny Protests in Russia case, the Board overturned Meta’s decision to remove a comment in which a supporter of the late Russian opposition leader Alexei Navalny called another user a “cowardly bot.”
Meta originally removed the comment for using the word “cowardly,” which was construed as a negative character claim. The Board found that, while removal of the content may have been consistent with a strict application of the Bullying and Harassment Community Standard, the enforcement of the policy failed to consider the wider context and disproportionately restricted freedom of expression.
As part of its decision, the Board recommended that whenever Meta removes content because of a negative character claim that is only a single word or phrase in a larger post, it should promptly notify users of that fact, so they can make changes and repost the material.
In response to this recommendation, when Meta’s automated systems detect that someone is about to publish content with a potential violation, the company now notifies users, so they have time to review it. This new alert provides an opportunity for people to delete and repost their content with edits, rather than it being potentially removed.
This change is already reaching millions of people. Over a 12-week period in 2023, more than 100 million pieces of content triggered these user notifications, 17 million of which were related to the Bullying and Harassment policy.
Content Moderation During Conflicts
Reliance on automation can be especially challenging when emergency situations put heightened stress on these systems. There is often an influx of content from regions experiencing conflict or crisis. This puts pressure on content moderation systems using AI and automation to identify violations, which risks increasing the rate of enforcement errors.
Meta’s automated classification systems (classifiers) use a variety of features when determining what action to take on content, including scoring on the probability of a violation, severity of the potential violation and virality of the content. In the Board’s first expedited decisions in 2023 about the Israel-Gaza conflict, the Board overturned Meta’s original decision to remove two posts from its platforms.
As part of its initial response to the conflict, Meta temporarily lowered the confidence thresholds for its classifiers that identify and remove content violating its Violent and Graphic Content, Hate Speech, Violence and Incitement, and Bullying and Harassment policies. The temporary measures applied to content originating in Israel and Gaza across all languages.
This meant that Meta used its automated tools to aggressively remove content that might even be slightly likely to violate its policies. While this reduced the likelihood that Meta would fail to remove violating content that might otherwise evade detection, it also led to drastic removal of non-violating content related to the conflict.
The Al-Shifa Hospital case, which focused on content containing video footage of a strike during Israeli military operations in Gaza, showed how a lack of human oversight during crisis response can lead to the incorrect removal of speech that may be of significant public interest. The initial decision to remove this content and the rejection of the user’s appeal were taken automatically based on a classifier score, without any human review.
Another expedited case, which involved a video showing Hostages Kidnapped From Israel during the October 7 terrorist attack by Hamas, highlighted concerns with content demotion. After the Board identified this case, Meta reversed its original decision to remove the post and restored it with a “mark as disturbing” warning screen. This restricted the visibility of the content to people over the age of 18 and removed it from recommendations to other Facebook users.
Removing content from recommendation systems means reducing the reach it would otherwise get. Demoting or applying other kinds of ‘soft actions’ to these types of posts, which have a public interest and are meant to call attention to human rights abuses, may not be a necessary or proportionate restriction on freedom of expression. This also calls into question the opacity of decisions to demote certain posts, made without explanation and in a non-transparent manner.
These cases underscore that platforms need to have a coherent and transparent approach to content moderation during conflicts. Social media companies cannot afford to improvise the rules during a moment of crisis. A lack of transparency around decision making can have a chilling effect on people who may fear their content will be removed and their account penalized if they make a mistake.
Automatic Content Enforcement Systems
Meta’s Media Matching Service banks, which are a type of automatic content enforcement system, are essentially repositories of content on which Meta has already made a moderation decision. These libraries of content – the “banks” – automatically identify images and videos already designated by human reviewers as either violating or not violating content policies, and act on the subsequent content based on the rules of that bank.
In the Board’s Colombian Police Cartoon case, the Board overturned Meta’s original decision to remove a Facebook post of a cartoon depicting police violence in Colombia. The cartoon was wrongly added by a human reviewer to Meta’s Media Matching Service bank, which led to a mass and disproportionate removal of the image from the platform. The Board found that 215 users appealed these removals, with 98% of those being successful. Such a high rate of overturn should have triggered a review, but Meta still did not remove the cartoon from this bank until the case came to the Board.
This case shows how automatic content removal systems can amplify the impact of incorrect decisions made by individual human reviewers. The stakes of mistaken additions to such systems are especially high when, as in this case, the content consists of political speech meant as a protest against government actors.
Conclusion
Social media companies are heavily reliant on AI and automated systems. Relevant reports from recent years show a massive increase in the amount of content automatically detected and removed from digital platforms. So far, the most common tools still sometimes fail to account for context and do not always provide detailed reasoning for why content was removed.
New generative AI models present major potential improvements in the ability to automatically identify violations of specific policy lines. It is possible that new generative AI tools will be better able to interpret the meaning of content and explain enforcement actions to users. But there is much work to be done to understand biases and errors in these systems to develop adequate oversight processes.
Although social media companies have signaled responsiveness to AI ethics concerns and challenges associated with generative AI, they must clearly articulate how they intend to align their development of and responses to new AI technologies with their responsibilities to respect human rights. Importantly, rigorous third-party accountability remains essential, including on major issues like addressing systemic risks to free expression, data access enabling evaluation of how accurately content moderation systems are working in general (beyond specific content cases), and transparency around penalties like content demotions or “shadow banning.”
Acknowledgements
This paper was written by a working group of Oversight Board members.